목록전체 글 (431)
mojo's Blog
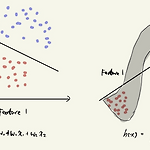
The Basic Concept of Logistic Regression ※ Problems in Simple Classification Models It is impossible to find a linear classifier in some cases. The loss function is not differential! 위 사진에서 왼쪽같은 경우는 Simple classification 모델을 통해 분류하는 것이 가능하다. 하지만 오른쪽은 어떤 선을 긋더라도 두 개의 클래스를 분류할 수 없는 경우가 존재한다. 이러한 케이스에서 Simple classification 을 통해 문제를 해결할 수 없다. ※ Probabilistic View for a Linear Classifier What if we ..
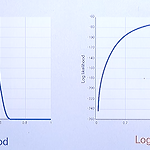
※ Estimation in Statistics Use sample statistics to estimate population parameters. -> E.g., Sample means are used to estimate population means. A point estimate of a population parameter is a single value of a statistic. An interval estimate is defined by two numbers, between which a population parameter is said to lie. - \(a < x < b\) is an interval estimate of the population mean \(\mu \). 일반..
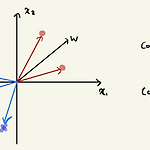
※ Example: Linear Regression Fitting a linear model with a set of variables \(x_{0}, x_{1}, ..., x_{d}\) \(f(x) = w_{0}x_{0} + w_{1}x_{1} + ... + w_{d}x_{d} \) Age and systolic blood pressure (SBP) 위 사진은 나이와 실제 blood pressure 혈압과의 관계를 표현한 데이터이다. 나이에 따라서 혈압이 오르는지 않오르는지 살펴보는 예제이다. 둘의 관계를 \(f(x)\) 와 같은 선형 함수로 표현이 된다고 가정한다. 이때, x는 age 밖에 존재하지 않으므로 \(f(x) = w_{1}x + w_{0}\) 의 모양으로 볼 수 있다. normal equa..
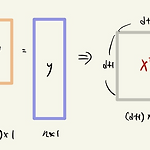
※ Regression Models Modeling the relationship between one or more explanatory variables \(x\) and a dependent variable \(y\) Widely used for predicting and forecasting continuous values x는 하나의 값으로 표현되거나 여러 개의 변수로 구성된 경우가 존재한다. y는 연속적인 값, 즉 실수형의 데이터로 표현된다. 회귀모델은 보통 날씨 예측이나 온도 예측, 가격 예측 등의 모델에서 활용될 수 있다. x가 한개인 경우 Simple, 2개 이상의 변수로 구성되어 있는 경우 Multiple 로 분류된다. 그리고 Simple, Multiple 에서 선형, 비선형으로 구성된..
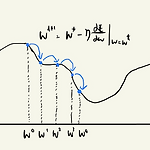
※ Challenges of Optimization Problem Finding minimum by closed form is often difficult or impossible. Quadratic functions in many variables - System of equations for partial derivatives may be ill-conditioned. m개의 변수보다 더 많은 파라메터를 가진 경우 기본적으로 analytic solution 을 구하는 것이 불가능하다. Other convex functions - Global minimum exists, but there is no closed form solution. convex function은 위와 같이 웅덩이 모양의 볼록 함수..
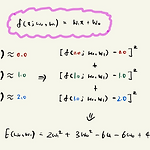
※ Three steps in Supervised Learning 1. 데이터를 수집하는 단계(Labelled Data) x와 y의 쌍 (x, y) 으로 구성된 데이터를 구축 x에 대응되는 y라는 레이블을 수집하는 labeling 작업을 거친다. 2. 모델 선택 단계(Model with tuneable parameters) 모델은 학습하고자 하는 parameter가 존재한다. parameter를 학습하는 방법은 주어진 x, y의 숨겨진 관계를 찾는 최적의 parameter를 찾는 과정이다. 3. 알고리즘 최적화(Optimization algorithm) x와 y 관계를 잘 표현하는 cost function 또는 error function 를 최적화하는 방향으로 우리가 원하는 parameter를 결정한다...